Plenary LectureS
Plenary Lectures will take place at the Auditorium of the Hotel Royal Continental (Continental building); they may also be followed in streaming at the Aula Magna of Partenope Congress Center.
ECC19 will feature the following five plenary speakers.
Day | Time | Title | Speaker | Affiliation |
Wed, June 26th | 8:30-9:30 | Learning and forecasts in autonomous systems | Francesco Borrelli | University of California, Berkeley |
Wed, June 26th | 16:00-17:00 | Optimal control at large | John Lygeros | ETH Zürich |
Thu, June 27th | 8:30-9:30 | Controlling complex networks | Raissa D’Souza | University of California, Davis |
Thu, June 27th | 16:00-17:00 | Airborne Wind Energy, data-based predictive control and the challenges of real-world autonomous systems | Lorenzo Fagiano | Politecnico di Milano |
Fri, June 28th | 8:30-9:30 | Optimization in networkland: Challenges and opportunities of distributed methods | Giuseppe Notarstefano | University of Bologna |
Abstracts of the lectrues and biosketch of the speakers are detailed below.
Learning and forecasts in autonomous systems
Scheduling: June 26th, 2019. Room: C-0-Auditorium (streaming at P-1-Aula Magna) . Time: 8:30-9:30.
Abstract. The complexity of modern autonomous systems has grown exponentially in the past decade. Today’s control engineers need to deliver high performance autonomy which is safe despite environment uncertainty, is able to effectively interact with humans, and improves system performance by using data processed on local and remote computing platforms.
Employing predictions of system dynamics, human behavior and environment components can facilitate such task. In addition, historical and real-time data can be used to bound forecasts uncertainty, learn model parameters and allow the system to adapt to new tasks.
Our research over the past decade has focused on control design for autonomous systems which systematically incorporate predictions and learning. In this talk I will first provide an overview of the theory and tools that we have developed for the designing of learning predictive controllers. Then, I will focus on recent results that use data to efficiently formulate stochastic control problems which autonomously improve performance in iterative tasks. Throughout the talk I will focus on autonomous cars to motivate our research and show the benefits of the proposed techniques.
More info on: www.mpc.berkeley.edu
Francesco Borrelli
Howard Penn Brown Professor
Dept. of Mechanical Engineering
University of California, Berkeley
Francesco Borrelli received the `Laurea’ degree in computer science engineering in 1998 from the University of Naples `Federico II’, Italy. In 2002 he received the PhD from the Automatic Control Laboratory at ETH-Zurich, Switzerland. He is currently a Professor at the Department of Mechanical Engineering of the University of California at Berkeley, USA. He is the author of more than one hundred fifty publications in the field of predictive control. He is author of the book Predictive Control published by Cambridge University Press, the winner of the 2009 NSF CAREER Award and the winner of the 2012 IEEE Control System Technology Award. In 2016 he was elected IEEE fellow. In 2017 he was awarded the Industrial Achievement Award by the International Federation of Automatic Control (IFAC) Council.
Since 2004 he has served as a consultant for major international corporations. He was the founder and CTO of BrightBox Technologies Inc, a company focused on cloud-computing optimization for autonomous systems. He is the co-director of the Hyundai Center of Excellence in Integrated Vehicle Safety Systems and Control at UC Berkeley. His research interest are in the area of model predictive control and its application to automated driving and energy systems.
Optimal control at large
Scheduling: June 26th, 2019. Room: C-0-Auditorium (streaming at P-1-Aula Magna) . Time: 16:00-17:00.
Abstract. Optimal control is an attractive framework for addressing complex control tasks, as it offers the promise of determining the best possible action given the dynamic and other constraints of the system. With some notable exceptions, however, optimal control problems are notoriously difficult to solve even for moderate size systems. It is known that many optimal control problems encoded as dynamic programs can equivalently be characterised through the solution of linear programs. Replacing the (often infinite) linear program by a simpler (finite) counterpart leads to methods for approximating the solution of the original optimal control problem, in the spirit of Approximate Dynamic Programming. In this talk we outline such an approach to approximate optimal control and discuss how results in randomised optimisation can be leveraged to derive bounds on the errors incurred in the process. The approach also offers a vista towards data driven control, as in some cases the approximation can be carried out using sample paths of the system evolution, bypassing the need to explicitly know or identify the dynamic constraints.
John Lygeros
Professor
Dept. of Information Technology and Electrical Engineering
ETH Zürich
John Lygeros completed a B.Eng. degree in electrical engineering in 1990 and an M.Sc. degree in Systems Control in 1991, both at Imperial College of Science Technology and Medicine, London. In 1996 he obtained a Ph.D. degree from the Electrical Engineering and Computer Sciences Department, University of California, Berkeley. After a series of postdoctoral researcher appointments, in 2000 he joined the Department of Engineering, University of Cambridge, U.K. as a University Lecturer. Between 2003 and 2006 he was an Assistant Professor at the Department of Electrical and Computer Engineering, University of Patras, Greece. In July 2006 he joined the Automatic Control Laboratory at ETH Zurich, where he is currently serving as a Full Professor of Computation and Control and the Head of the laboratory. His research interests include modelling, analysis, and control of hierarchical, hybrid, and stochastic systems, with applications to biochemical networks, automated highway systems, air traffic management, and energy systems. John Lygeros is a Fellow of the IEEE, and a member of the IET and the Technical Chamber of Greece; since 2013 he is serving as the Treasurer of the International Federation of Automatic Control.
Controlling complex networks
Scheduling: June 27th, 2019. Room: C-0-Auditorium (streaming at P-1-Aula Magna) . Time: 8:30-9:30.
Abstract. Networks are at the core of modern society, spanning physical, biological and social systems. Each distinct network is typically a complex system, shaped by the collective action of individual agents and displaying emergent behaviors. Moreover, interactions across networks can lead to unanticipated cascading failures and novel phase transitions. How will we control these massive, self-organizing and typically non-linear systems? Three main threads include structural controllability, control of nonlinear systems, and control and influence in social systems. Here we will survey progress in these areas, highlighting testbeds at three scales. Probing into nonlinear dynamics, we study both theoretically and empirically the attractor space of synchronization for a ring of reactively coupled nanoelectromechanical oscillators. At the mega-scale of critical infrastructure, our focus is on understanding interdependence between power, gas and water networks and leveraging this for resilience and restoration efforts. Finally at the scale of social systems we study the multilayered interactions found in macaque monkey societies, including aggression, grooming, policing and huddling networks.
Raissa D’Souza
Professor
Dept. of Computer Science
University of California, Davis
Raissa D’Souza is Professor of Computer Science and of Mechanical Engineering at the University of California, Davis, as well as an External Professor at the Santa Fe Institute. She received a PhD in Statistical Physics from MIT in 1999, then was a postdoctoral fellow at Bell Laboratories followed by the Theory Group at Microsoft Research. Her interdisciplinary work on network theory spans the fields of statistical physics, theoretical computer science and applied math, and has appeared in journals such as Science, PNAS, and Physical Review Letters. She is a Fellow of the American Physical Society, the recipient of the 2017 Outstanding mid-career research award for the UCD College of Engineering, and serves on the editorial board of numerous mathematics and physics journals. She has organized key scientific meetings like NetSci 2014, and was a member of the World Economic Forum’s Global Agenda Council on Complex Systems. She served as President of the Network Science Society, 2015-18, and is Outgoing President 2018-19.
Airborne Wind Energy, data-based predictive control and the challenges of real-world autonomous systems
This plenary is proposed by the 2019 EUCA Award winner.
Scheduling: June 27th, 2019. Room: C-0-Auditorium (streaming at P-1-Aula Magna) . Time: 16:00-17:00.
Abstract. Airborne Wind Energy (AWE) is an innovative concept that exploits autonomous tethered aircrafts to harvest wind energy. Thanks to its flexibility and predicted low capital cost, AWE promises a strong complementarity with existing renewables and a significant increase of the total share of renewables in our energy mix. Conceived in the late ‘70s and pioneered experimentally starting around 2004, AWE systems represent today a growing R&D sector, with clear technology convergence and industrialization processes in place. Yet, the road to commercialization is still risky and presents important challenges, many in the area of automation and control engineering. As an example, these systems will have to operate with minimal supervision, fully autonomously for decades, in a highly uncertain and harsh environment, carrying out repeated, feedback-stabilized trajectories. This talk will present the main aspects of AWE and the milestones achieved, provide an overview of the sector, and describe the challenges ahead. These challenges motivate recent research in the area of data-based predictive control of uncertain systems, which will be reviewed as well.
Lorenzo Fagiano
Associate Professor
Dip. di Elettronica, Informazione e Bioingegneria
Politecnico di Milano
Lorenzo Fagiano received the M.Sc. degree in Automotive Engineering in 2004 and the Ph.D. degree in Information and Systems Engineering in 2009 from Politecnico di Torino, Italy. In 2005 he was with Fiat Research Centre, Torino, Italy. From 2010 to 2013 he held positions as Marie Curie Fellow at the Politecnico di Torino, the University of California at Santa Barbara, and ETH Zurich. From 2013 to 2016 he was scientist and senior scientist at ABB Switzerland, Corporate Research. Lorenzo Fagiano is currently associate professor of automation and control engineering at the Politecnico di Milano, Italy. His research interests include constrained estimation and control, set membership methods, and applications to industrial, robotic and energy systems. He serves as associate editor of the IEEE Transactions of Control Systems Technology since 2015. He is recipient of the 2011 IEEE Transactions on Systems Technology Outstanding Paper Award, of the 2010 ENI award “Debut in Research” prize, and of two Marie Curie individual fellowships. He is co-founder of two start-up companies, in the fields of airborne wind energy and multicopter drones for civil applications.
Optimization in networkland: Challenges and opportunities of distributed methods
Scheduling: June 28th, 2019. Room: C-0-Auditorium (streaming at P-1-Aula Magna) . Time: 8:30-9:30.
Abstract. Optimization is a building block for the solution of several problems in estimation, learning, decision and control. Nowadays, due to the massive and ubiquitous presence of smart devices with sensing and control capabilities, large-scale optimization problems arise in these areas, so that powerful computing architectures are required to solve them. On the other side, smart networks of connected devices can be seen as extremely powerful, but completely unstructured, “supercomputing” infrastructures, in which nodes have only a partial knowledge of the optimization problem to solve. Distributed optimization aims at exploiting such an opportunity by developing novel methods, based on local computation and communication, allowing network processors to cooperatively solve the global optimization problem without relying on any central unit. In this talk I will start introducing some classes of structured optimization problems motivated by learning and control applications in cyber-physical networks, and show key challenges that arise in a distributed computation framework. Then, I will present novel distributed optimization methods to address some of these challenges as: asynchronous and possibly unreliable communication, a large number of decision variables and/or constraints, and nonconvex problems as, e.g., mixed-integer programs.
Giuseppe Notarstefano
Professor
Dept. of Electrical, Electronic, and Information Engineering
G. Marconi at Alma Mater Studiorum
Università di Bologna
Giuseppe Notarstefano is a Professor in the Department of Electrical, Electronic, and Information Engineering G. Marconi at Alma Mater Studiorum Università di Bologna. He was Associate Professor (from June ‘16 to June ‘18) and previously Assistant Professor, Ricercatore, (from February ‘07) at the Università del Salento, Lecce, Italy. He received the Laurea degree “summa cum laude” in Electronics Engineering from the Università di Pisa in 2003 and the Ph.D. degree in Automation and Operation Research from the Università di Padova in 2007. He has been visiting scholar at the University of Stuttgart, University of California Santa Barbara and University of Colorado Boulder. His research interests include distributed optimization, cooperative control in complex networks, applied nonlinear optimal control, and trajectory optimization and maneuvering of aerial and car vehicles. He serves as an Associate Editor for IEEE Transactions on Automatic Control, IEEE Transactions on Control Systems Technology and IEEE Control Systems Letters. He is also part of the Conference Editorial Board of IEEE Control Systems Society and EUCA. He is recipient of an ERC Starting Grant 2014.
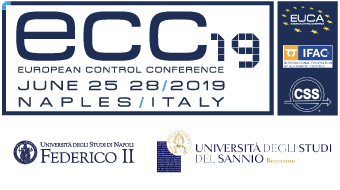
secretariat:
via dei mille 16
80121 naples (italy)
for accommodation:
administrative queries:
for technical info:

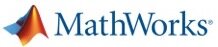
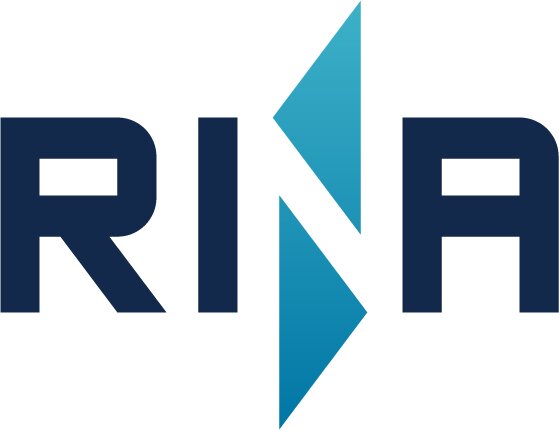

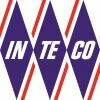
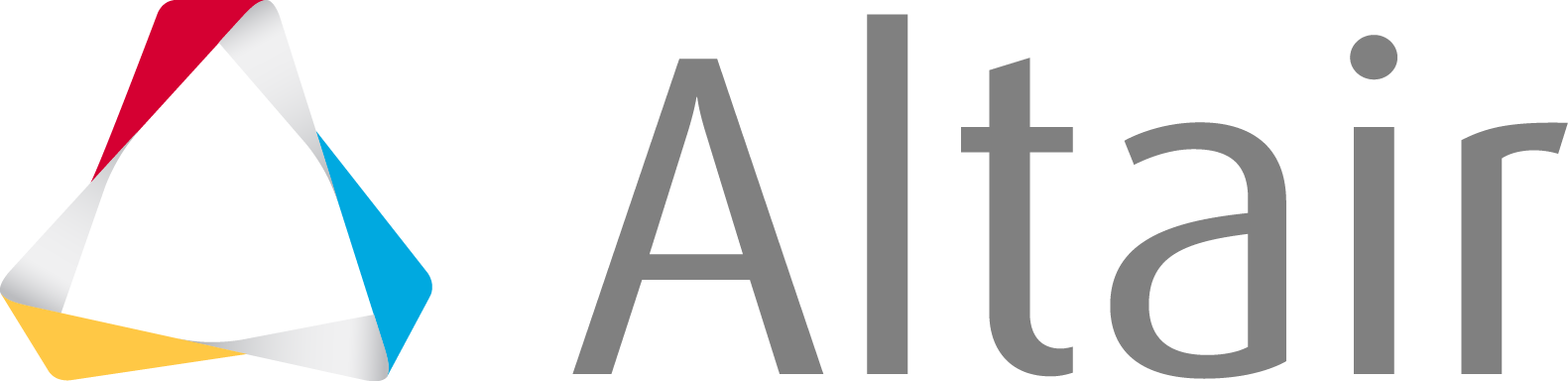
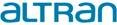
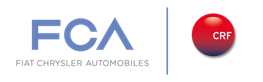
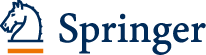

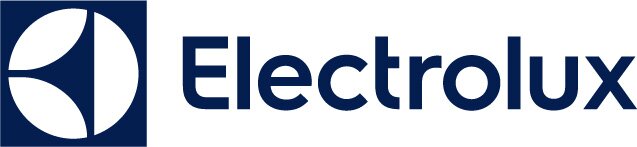